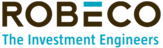
免責聲明
1. 一般事項
請細閱以下資料。
此網站由Robeco Hong Kong Limited(「荷寶」)擬備及刊發,荷寶是獲香港證券及期貨事務監察委員會發牌從事第1類(證券交易)、第4類(就證券提供意見)及第9類(資產管理)受規管活動的企業。荷寶不持有客戶資產,並受到發牌條件所規限。荷寶在擴展至零售業務之前,必須先得到證監會的批准。本網頁未經證券及期貨事務監察委員會或香港的任何監管當局審閱。
2. 風險披露聲明
Robeco Capital Growth Funds以其特定的投資政策或其他特徵作識別,請小心閱讀有關Robeco Capital Growth Funds的風險:
部份基金可涉及投資、市場、股票投資、流動性、交易對手、證券借貸及外幣風險及小型及/或中型公司的相關風險。
部份基金所涉及投資於新興市場的風險包括政治、經濟、法律、規管、市場、結算、執行交易、交易對手及貨幣風險。
部份基金可透過合格境外機構投資者("QFII")及/或 人民幣合格境外機構投資者 ("RQFII")及/或 滬港通計劃直接投資於中國A股,當中涉及額外的結算、規管、營運、交易對手及流動性風險。
就分派股息類別,部份基金可能從資本中作出股息分派。股息分派若直接從資本中撥付,這代表投資者獲付還或提取原有投資本金的部份金額或原有投資應佔的任何資本收益,該等分派可能導致基金的每股資產淨值即時減少。
部份基金投資可能集中在單一地區/單一國家/相同行業及/或相同主題營運。 因此,基金的價值可能會較為波動。
部份基金使用的任何量化技巧可能無效,可能對基金的價值構成不利影響。
除了投資、市場、流動性、交易對手、證券借貸、(反向)回購協議及外幣風險,部份基金可涉及定息收入投資有關的風險包括信貨風險、利率風險、可換股債券的風險、資產抵押證券的的風險、投資於非投資級別或不獲評級證券的風險及投資於未達投資級別主權證券的風險。
部份基金可大量運用金融衍生工具。荷寶環球消費新趨勢股票可為對沖目的及為有效投資組合管理而運用金融衍生工具。運用金融衍生工具可涉及較高的交易對手、流通性及估值的風險。在不利的情況下,部份基金可能會因為使用金融衍生工具而承受重大虧損(甚至損失基金資產的全部)。
荷寶歐洲高收益債券可涉及投資歐元區的風險。
投資者在Robeco Capital Growth Funds的投資有可能大幅虧損。投資者應該參閱Robeco Capital Growth Funds之銷售文件內的資料﹙包括潛在風險﹚,而不應只根據這文件內的資料而作出投資。
3. 當地的法律及銷售限制
此網站僅供“專業投資者”進接(其定義根據香港法律《證券及期貨條例》(第571章)和/或《證券及期貨(專業投資者)規則》(第571D章)所載)。此網站並非以在禁止刊發或提供此網站(基於該人士的國籍、居住地或其他原因)的任何司法管轄區內的任何人士為對象。受該等禁例限制的人士或並非上述訂明的人士不得登入此網站。登入此網站的人士需注意,他們有責任遵守所有當地法例及法規。一經登入此網站及其任何網頁,即確認閣下已同意並理解以下使用條款及法律資料。若閣下不同意以下條款及條件,不得登入此網站及其任何網頁。
此網站所載的資料僅供資料參考用途。
在此網站發表的任何資料或意見,概不構成購買、出售或銷售任何投資,參與任何其他交易或提供任何投資建議或服務的招攬、要約或建議。此網站所載的資料並不構成投資意見或建議,擬備時並無考慮可能取得此網站的任何特定人士的個別目標、財務狀況或需要。投資於荷寶產品前,必須先細閱相關的法律文件,例如管理法規、基金章程、最新的年度及半年度報告,所有該等文件可於www.robeco.com/hk/zh免費下載,亦可向荷寶於香港的辦事處免費索取。
4. 使用此網站
有關資料建基於特定時間適用的若干假設、資料及條件,可隨時更改,毋需另行通知。儘管荷寶旨在提供準確、完整及最新的資料,並獲取自相信為可靠的資料來源,但概不就該等資料的準確性或完整性作出明示或暗示的保證或聲明。
登入此網站的人士需為其資料的選擇和使用負責。
5. 投資表現
概不保證將可達到任何投資產品的投資目標。並不就任何投資產品的表現或投資回報作出陳述或承諾。閣下的投資價值可能反覆波動。荷寶投資產品的資產價值可能亦會因投資政策及/或金融市場的發展而反覆波動。過去所得的業績並不保證未來回報。此網站所載的往績、預估或預測不應被視為未來表現的指示或保證,概不就未來表現作出任何明示或暗示的陳述或保證。基金的表現數據以月底的交易價格為基礎,並以總回報基礎及股息再作投資計算。對比基準的回報數據顯示未計管理及/或表現費前的投資管理業績;基金回報包括股息再作投資,並以基準估值時的價格及匯率計算的資產淨值為基礎。
投資涉及風險。往績並非未來表現的指引。準投資者在作出任何投資決定前,應細閱相關發售文件所載的條款及條件,特別是投資政策及風險因素。投資者應確保其完全明白與基金相關的風險,並應考慮其投資目標及風險承受程度。投資者應注意,基金股份的價格及收益(如有)可能反覆波動,並可能在短時間內大幅變動,投資者或無法取回其投資於基金的金額。若有任何疑問,請諮詢獨立財務及有關專家的意見。
6. 第三者網站
本網站含有來自第三方的資料或第三方經營的網站連結,而其中部分該等公司與荷寶沒有任何聯繫。跟隨連結登入任何其他此網站以外的網頁或第三方網站的風險,應由跟隨該連結的人士自行承擔。荷寶並無審閱此網站所連結或提述的任何網站,概不就該等網站的內容或所提供的產品、服務或其他項目作出推許或負上任何責任。荷寶概不就使用或依賴第三方網站所載的資料而導致的任何虧損或損毀負上法侓責任,包括(但不限於)任何虧損或利益或任何其他直接或間接的損毀。 此網站以外的網頁或第三方網站皆旨在作參考之用。
7. 責任限制
荷寶及(潛在的)其他網站資料供應商概不就此網站內容或其所載的資料或建議負責,而該等內容、資料或建議可予更改,毋需另行通知。
荷寶並無責任確保及保證此網站的功能將不受干擾或並無失誤。荷寶概不就有關荷寶(交易)服務電郵訊息的後果承擔任何責任,該等電郵訊息可能無法接收或發出、損毀、不正確接收或發出或並無準時接收或發出。
荷寶亦不就因登入及使用此網站而可能導致的任何虧損或損毀負責。
8. 知識產權
所有版權、專利、知識產權和其他財產,以及有關此網站資料的授權均由荷寶持有及獲取。該等權利不會轉授予查閱有關資料的人士。
9. 私隠
荷寶保證將會根據現行的資料保障法例,以保密方式處理登入此網站的人士的數據。除非荷寶需按法律責任行事,否則在未經登入此網站的人士許可,不會向第三方提供該等數據。 請於我們的私隱及Cookie政策 中查找更多詳情。
10. 適用法律
此網站受香港法律監管及據此解釋。因此網站導致或有關此網站的所有爭議應交由香港法庭作出專有裁決。
如果您已閱讀並理解本頁並同意上述免責聲明以及同意荷寶收集和使用您的個人資料,用於私隱及Cookie政策 所列的收集和使用個人資料的目的(包括用於直接推廣荷寶的產品或服務),請點擊“我同意”按鈕。否則,請點擊“我不同意”離開本網站。
市場觀點
對專業投資者而言,了解市場走向及其影響可說是最艱巨的任務。他們必須對投資市場有深入的研究和見解,因此我們在這個領域不斷投放大量精力。我們對自己的專業領域有深入的了解,並積極與您分享我們的觀點。因為只有分享知識,才能促進社會繁榮。
746 文章
焦點主題
內容類型
地區
策略