In the capital asset pricing model (CAPM), beta is used to describe how the returns of a particular stock (or portfolio) are related to those of the market. It is defined as the correlation between a specific stock (or portfolio) and the market, multiplied by the relative volatility of the stock (or portfolio) versus the market.
Beta is, however, an unobserved characteristic that needs to be estimated. Thus, finding the best way to forecast the beta of securities is an important consideration for academics and practitioners. But more importantly, dealing with the accompanying estimation error is a crucial step in the process. Robeco researchers tackled these issues in an academic paper1 that focused on beta estimations using shrinkage techniques, by looking at the US stock market from January 1963 to December 2017.
Beta shrinkage lowers estimation errors
For the study, the authors assumed that correlations and relative volatilities are independent of each other, such that beta expectations are equal to the product of these two estimated components. To examine which approach best forecasts beta, they analyzed a range of methods, from those that shrink the beta in its entirety, to others that reduce estimation errors in correlations and relative volatilities to their cross-sectional averages separately.
To investigate the effect of implementing shrinkage on correlations and relative volatilities, they looked at the resulting mean squared errors (MSE) of the beta estimates when correlations and relative volatilities were shrunk at different levels. This ranged from a shrinkage factor of 0 to 1 for both parameters.
The analysis confirmed that the largest estimation error – or highest MSE – occurred when no shrinkage was implemented. Meanwhile, a two-parameter approach – that reduced correlations (0.5 shrinkage factor) more than relative volatilities (0.2 shrinkage factor) – produced the best outcome (or lowest MSE). Moreover, one-parameter settings generally delivered suboptimal results.
The researchers also scrutinized stocks that had the most dissimilar beta predictions when the no shrinkage and two-parameter shrinkage approaches were applied. This comparison was also made between the related estimates of the one-parameter shrinkage and two-parameter shrinkage settings. Thereafter, the stocks were ranked on the differences in beta forecasts between these methods and allocated to 10-decile portfolios. The average ex-ante beta estimates for these portfolios were then compared with their realized betas over the full sample period.
The results revealed that the two-parameter shrinkage method had lower forecasting errors than the other two approaches. Indeed, Figure 1 shows the ex-post absolute beta forecast error for both the no shrinkage and two-parameter shrinkage settings for each of the 10-decile portfolios, while the horizontal axis reflects the differences in ex-ante average beta estimates between the two methods.
Figure 1 | Decile portfolios sorted on beta estimate differences between no shrinkage and two-parameter shrinkage methods
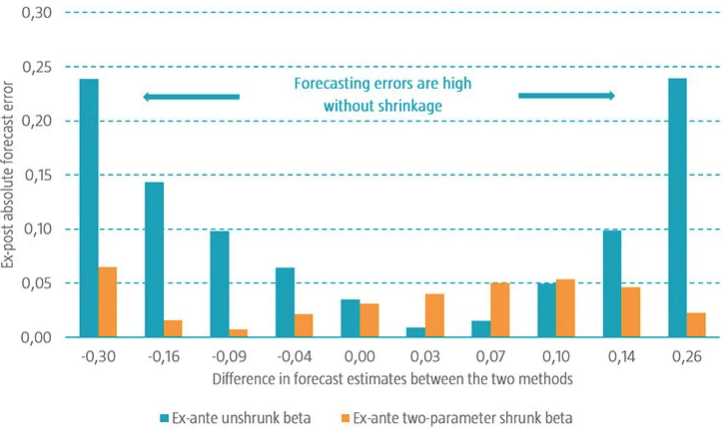
Source: Blitz, D., Swinkels, L., Ūsaitė, K., and Van Vliet, P., December 2021, “Shrinking beta”, SSRN working paper.
“
Improved ex-ante beta estimates at the individual stock level led to lower ex-post portfolio betas
Shrunk betas result in less risky portfolios
The authors also assessed whether these improved ex-ante beta estimates at the individual stock level led to lower ex-post portfolio betas. They ranked stocks on their historical beta estimates and allocated them to 10-decile portfolios. The same exercise was performed using the two-parameter shrinkage beta forecasts. In addition, a long-short strategy based on a long position in the D1 portfolio (lowest beta estimates) and short position in D10 portfolio (highest beta estimates) was constructed.
For the strategies based on historical beta estimates, the results showed that the D1 portfolio had an average excess return of 7.26%, volatility of 11.04%, Sharpe ratio of 0.66, and market beta of 0.45. On the other hand, the D10 portfolio also had an average return of 7.26%, but a volatility of 32.59%, Sharpe ratio of 0.22, and a market beta of 1.65. Meanwhile, the long-short portfolio exhibited an alpha of 10.14% relative to the CAPM.
For the strategies based on the two-parameter shrinkage beta estimates, the D1 portfolio had a lower ex-post beta (from 0.45 to 0.42) and a lower realized volatility (from 11.04% to 10.79%). In addition, the D10 portfolio had a higher volatility and a higher ex-post beta. This also indicates that risk can be better predicted on an overall portfolio level. Finally, the long-short portfolio delivered a CAPM alpha that was almost 2% higher (from 10.14% to 11.87%) and that was statistically significant.
Comparing low beta and Low Volatility portfolios
In their paper, the researchers found that beta predictions were more accurate when correlations were shrunk more to their cross-sectional average than relative volatilities. For portfolio construction purposes, these results could be seen as a reason to allocate less weight to estimated correlations than forecasted relative volatilities. To assess this notion, the authors took a different approach and formed portfolios based on either two-parameter shrunk betas, volatility, or a combination of these two variables. This analysis gave them insight on the effect of including correlations alongside volatilities, thereby connecting the literature of low beta and low volatility investing.
When different portfolio combinations based on shrunk beta estimates and volatilities were evaluated, the results revealed that including correlation significantly reduced the volatility of a low-risk portfolio. Therefore, this indicates that correlation is indeed an important element to consider within a low volatility portfolio.
“
An equally weighted combination of unshrunk betas and volatility are most effective in reducing volatility
However, sorting stocks into portfolios based on their conventional betas gives too much weight to the correlation, which is less accurately forecasted. This can be reduced, however, by shrinking correlations more to their cross-sectional average than volatilities, or by combining conventional betas with pure volatility estimates. This latter approach of equally combining beta and volatility is less complex because there is no need to estimate shrinkage parameters.
This concept is illustrated in Figure 2, which shows that the portfolios based only on conventional beta exhibit the highest realized volatility. Furthermore, it depicts that standalone volatility is a better predictor than standalone beta. Meanwhile, those that are based on two-parameter shrunk betas or use an equally weighted combination of unshrunk betas and volatility are most effective in reducing volatility.
Figure 2 | Comparing the realized volatilities of portfolios based on four different construction methods
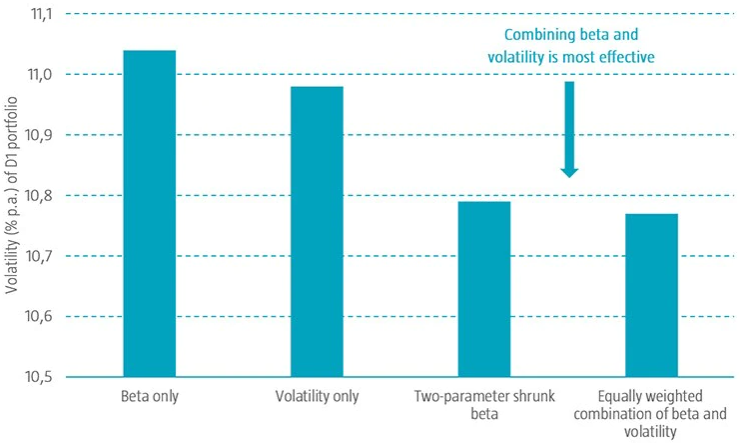
Source: Blitz, D., Swinkels, L., Ūsaitė, K., and Van Vliet, P., December 2021, “Shrinking beta”, SSRN working paper.
All in all, the results from this research paper indicate that correlations can help enhance the risk-return outcomes of low-risk portfolios. But since they are less accurately estimated than volatilities, they should be handled with care.
Read the full research paper重要事項
当資料は情報提供を目的として、Robeco Institutional Asset Management B.V.が作成した英文資料、もしくはその英文資料をロベコ・ジャパン株式会社が翻訳したものです。資料中の個別の金融商品の売買の勧誘や推奨等を目的とするものではありません。記載された情報は十分信頼できるものであると考えておりますが、その正確性、完全性を保証するものではありません。意見や見通しはあくまで作成日における弊社の判断に基づくものであり、今後予告なしに変更されることがあります。運用状況、市場動向、意見等は、過去の一時点あるいは過去の一定期間についてのものであり、過去の実績は将来の運用成果を保証または示唆するものではありません。また、記載された投資方針・戦略等は全ての投資家の皆様に適合するとは限りません。当資料は法律、税務、会計面での助言の提供を意図するものではありません。 ご契約に際しては、必要に応じ専門家にご相談の上、最終的なご判断はお客様ご自身でなさるようお願い致します。 運用を行う資産の評価額は、組入有価証券等の価格、金融市場の相場や金利等の変動、及び組入有価証券の発行体の財務状況による信用力等の影響を受けて変動します。また、外貨建資産に投資する場合は為替変動の影響も受けます。運用によって生じた損益は、全て投資家の皆様に帰属します。したがって投資元本や一定の運用成果が保証されているものではなく、投資元本を上回る損失を被ることがあります。弊社が行う金融商品取引業に係る手数料または報酬は、締結される契約の種類や契約資産額により異なるため、当資料において記載せず別途ご提示させて頂く場合があります。具体的な手数料または報酬の金額・計算方法につきましては弊社担当者へお問合せください。 当資料及び記載されている情報、商品に関する権利は弊社に帰属します。したがって、弊社の書面による同意なくしてその全部もしくは一部を複製またはその他の方法で配布することはご遠慮ください。 商号等: ロベコ・ジャパン株式会社 金融商品取引業者 関東財務局長(金商)第2780号 加入協会: 一般社団法人 日本投資顧問業協会