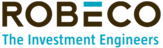
Important information
The Robeco Capital Growth Funds have not been registered under the United States Investment Company Act of 1940, as amended, nor or the United States Securities Act of 1933, as amended. None of the shares may be offered or sold, directly or indirectly in the United States or to any U.S. Person (within the meaning of Regulation S promulgated under the Securities Act of 1933, as amended (the “Securities Act”)). Furthermore, Robeco Institutional Asset Management B.V. (Robeco) does not provide investment advisory services, or hold itself out as providing investment advisory services, in the United States or to any U.S. Person (within the meaning of Regulation S promulgated under the Securities Act).
This website is intended for use only by non-U.S. Persons outside of the United States (within the meaning of Regulation S promulgated under the Securities Act who are professional investors, or professional fiduciaries representing such non-U.S. Person investors. By clicking “I Agree” on our website disclaimer and accessing the information on this website, including any subdomain thereof, you are certifying and agreeing to the following: (i) you have read, understood and agree to this disclaimer, (ii) you have informed yourself of any applicable legal restrictions and represent that by accessing the information contained on this website, you are not in violation of, and will not be causing Robeco or any of its affiliated entities or issuers to violate, any applicable laws and, as a result, you are legally authorized to access such information on behalf of yourself and any underlying investment advisory client, (iii) you understand and acknowledge that certain information presented herein relates to securities that have not been registered under the Securities Act, and may be offered or sold only outside the United States and only to, or for the account or benefit of, non-U.S. Persons (within the meaning of Regulation S under the Securities Act), (iv) you are, or are a discretionary investment adviser representing, a non-U.S. Person (within the meaning of Regulation S under the Securities Act) located outside of the United States and (v) you are, or are a discretionary investment adviser representing, a professional non-retail investor. Access to this website has been limited so that it shall not constitute directed selling efforts (as defined in Regulation S under the Securities Act) in the United States and so that it shall not be deemed to constitute Robeco holding itself out generally to the public in the U.S. as an investment adviser. Nothing contained herein constitutes an offer to sell securities or solicitation of an offer to purchase any securities in any jurisdiction. We reserve the right to deny access to any visitor, including, but not limited to, those visitors with IP addresses residing in the United States.
This website has been carefully prepared by Robeco. The information contained in this publication is based upon sources of information believed to be reliable. Robeco is not answerable for the accuracy or completeness of the facts, opinions, expectations and results referred to therein. Whilst every care has been taken in the preparation of this website, we do not accept any responsibility for damage of any kind resulting from incorrect or incomplete information. This website is subject to change without notice. The value of the investments may fluctuate. Past performance is no guarantee of future results. If the currency in which the past performance is displayed differs from the currency of the country in which you reside, then you should be aware that due to exchange rate fluctuations the performance shown may increase or decrease if converted into your local currency. For investment professional use only. Not for use by the general public.
Our insights
Understanding where markets are going, and the impact that has, is the hardest thing for every investment professional to do. It takes robust research and insight to develop the best understanding, and that’s why we put a lot of effort into it. Because only by sharing knowledge can we all prosper.
715 articles
Focus themes
Content type
Region
Strategies