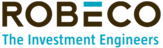
Disclaimer
The information contained in the website is solely intended for professional investors. Some funds shown on this website fall outside the scope of the Dutch Act on the Financial Supervision (Wet op het financieel toezicht) and therefore do not (need to) have a license from the Authority for the Financial Markets (AFM).
The funds shown on this website may not be available in your country. Please select your country website (top right corner) to view more information.
Neither information nor any opinion expressed on the website constitutes a solicitation, an offer or a recommendation to buy, sell or dispose of any investment, to engage in any other transaction or to provide any investment advice or service. An investment in a Robeco product should only be made after reading the related legal documents such as management regulations, prospectuses, annual and semi-annual reports, which can be all be obtained free of charge at this website and at the Robeco offices in each country where Robeco has a presence.
By clicking Proceed I confirm that I am a professional investor and that I have read, understood and accept the terms of use for this website.
Committed to performance
Who we are
Nice to meet you
As a global asset manager, we center our solutions around your needs. We’re driven by endless curiosity, rigorous research and a proven passion for sustainability, and we’ll guide you towards ground-breaking active investment strategies.
Interested in investing for today and tomorrow’s needs? Having our investments contribute to a healthy planet and society is our common goal. Let’s share the conversation: talk to one of our colleagues.
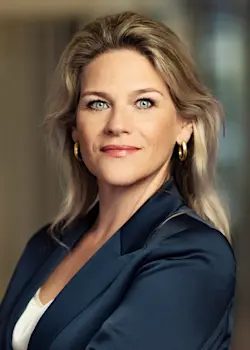
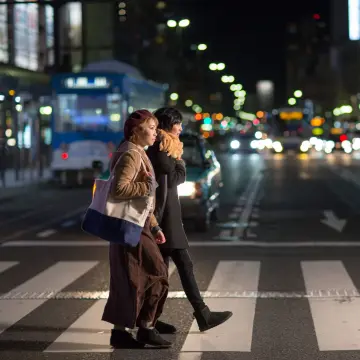
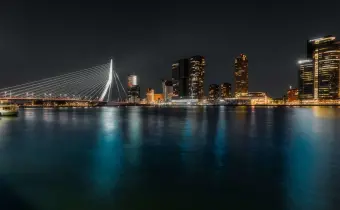
Opportunities
We are pleased to highlight some opportunities selected by our experts.
Active Quant
Fundamental equities
Credit investing
Value investing
Active Quant