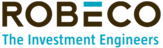
Disclaimer
The information contained in the website is solely intended for professional investors. Some funds shown on this website fall outside the scope of the Dutch Act on the Financial Supervision (Wet op het financieel toezicht) and therefore do not (need to) have a license from the Authority for the Financial Markets (AFM).
The funds shown on this website may not be available in your country. Please select your country website (top right corner) to view more information.
Neither information nor any opinion expressed on the website constitutes a solicitation, an offer or a recommendation to buy, sell or dispose of any investment, to engage in any other transaction or to provide any investment advice or service. An investment in a Robeco product should only be made after reading the related legal documents such as management regulations, prospectuses, annual and semi-annual reports, which can be all be obtained free of charge at this website and at the Robeco offices in each country where Robeco has a presence.
By clicking Proceed I confirm that I am a professional investor and that I have read, understood and accept the terms of use for this website.
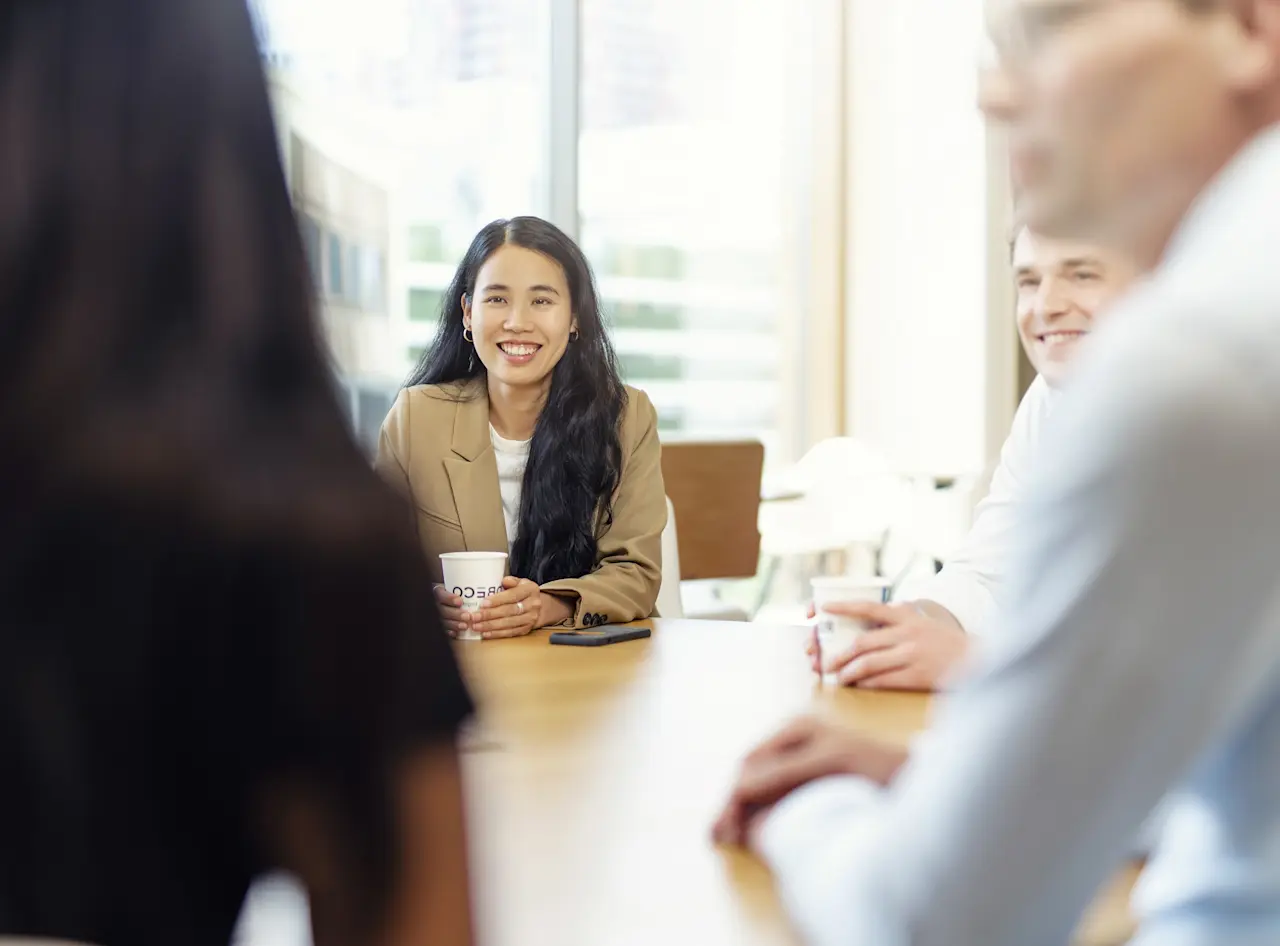
Job opportunities
Accelerate your career
Are you interested in working at Robeco? As a Dutch asset manager operating globally, Robeco combines the best of both worlds. We are one of the highest regarded international asset managers in the world and can offer you an equally rewarding career.
Working at Robeco
Joining Robeco means more than just a job – it’s an opportunity to unlock your full potential in a dynamic and collaborative environment. We’re dedicated to empowering our employees to thrive, fostering personal and professional growth at every step.
We strongly believe that our performance is the combination of both results and our behavior. We therefore invest in both your ability to get results and your ability to lead.
At Robeco, we want to grow every day: as a company and as an employer. To do better, we listen to and learn from society, our clients and – first and foremost – our colleagues.
Grow personally and professionally
Robeco Learning & Development actively promotes the development of Robeco’s employees with professional advice and offers several development activities. We aim to inspire and guide them so that they can perform to the best of their ability and make a valuable long-term contribution to our business strategy.
To facilitate this, Robeco offers a variety of development opportunities for its employees. Examples are training programs for people leaders, experts and young professionals, coaching & mentoring opportunities, specific professional qualification trainings and online learnings.
Diversity, Equity & Inclusion
Robeco is committed to promoting diversity, equity and inclusion among its employees. We consider this to be a core value in how we operate, recruit, build our strategy, and shape our culture.

Internship
Are you a student looking to work at the cutting edge of academia and practice? For anyone interested in finance, few things are more exciting than trying to beat global markets using state-of-the-art research. You can get a taste of it by joining our internship program.
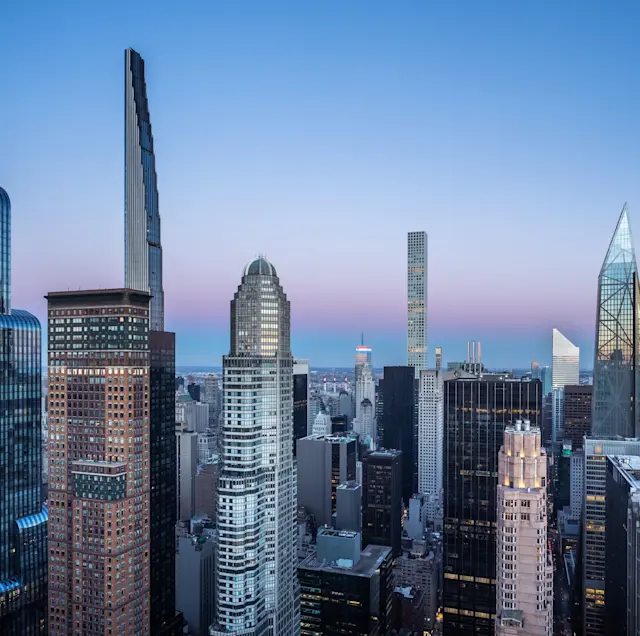