Not only is generative AI seen as a new companion for casual conversations, but as in other industries, finance practitioners are fascinated by the possibilities. However, the majority of the industry is still in the early stages of exploring its potential or beginning to integrate it into their workflows. Below we take a look at AI’s recent history and where we are today.
Then and now in AI
Researchers have relied on fundamental principles and mathematical derivations throughout history to make predictions and decisions. But the increased complexity of tasks today calls for new methods and algorithms to identify more intricate relationships between many variables. To this end, more advanced systems and algorithms have been developed to conduct heavy data-processing tasks and make human-like intelligent decisions: AI. In machine learning (ML), problems are solved using algorithms that can adapt, learn, and discover their own rules and relationships, while in natural language processing (NLP), computers quantify and comprehend textual and/or speech data.
The most recent development – generative AI models – encompass deep neural networks that learn the nature of the input training data to then generate new data that has similar characteristics. Well-known examples of generative AI models include GPT-4 for textual applications and DALL-E for image applications.
Figure 1: AI developments
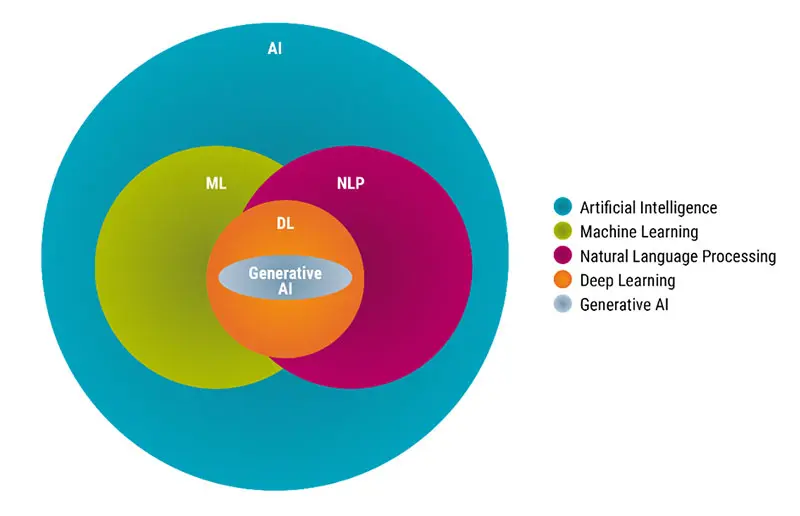
Source: Robeco
In field after field, AI techniques have made their way into various tasks such as facial recognition, internet searches, medical diagnosis, autonomous driving, or playing chess and Go. In this last example and indeed many other fields, machines have become fully autonomous and outperformed their human contenders.
ML developments in finance
While ML excels at many complex tasks, investment brings a whole new level of difficulty because it relates to predictions and decisions on the economy and financial markets. These are influenced by millions of human beings who are in turn influenced by a myriad of variables. The finance industry began adopting elements of AI as early as the 1990s, with varying degrees of success. For instance, consumer credit default probability and fraud detection models were among the first to use ML techniques. Conversely, the practical use of ML for stock selection and market timing beyond simple quantitative algorithms has only taken off in the last decade.
Three reasons stand out as to why asset management applications have adopted such techniques only recently. First, ML models require a massive amount of training data, which is more abundant in some fields than others. Secondly, finance is dynamic and adaptive unlike fields bound by rigid rules. This, coupled with the diverse reactions of investors to similar decisions at different times, adds complexity. Thirdly, stock price patterns are not obvious, otherwise, they would be quickly arbitraged away by smart market participants.
Nevertheless, there are many instances where machines can help humans in investment decisions, owing to the observation that “history never repeats itself, but it often rhymes.” While ML models do not have domain knowledge per se, the practitioners developing these algorithms do. As a result, ML models can be directed to learn the relevant features by going through many examples in the training data, with algorithms generally becoming more robust and accurate as machines are fed with more data.
ML applications in asset management
How can ML benefit asset management? Over the past few years, academic research has investigated its efficacy in stock and bond price prediction, as these models can identify complex patterns that might go unnoticed to the human eye, especially regarding asymmetries and interaction effects.
Short-term price reversal is a robust stock price pattern: When stocks experience a large price move over a short period (a couple of days), this price move tends to partially reverse over the next few days. Short-term reversal is a prominent feature in ML models predicting the cross-section of equity returns, often exhibiting interaction effects with other features.
Figure 2: Stock price pattern conditioned on the presence of recent news
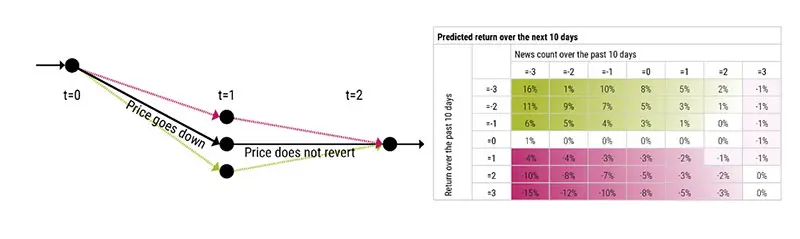
Source: ‘8 things you should know about next-gen quant’ Mike Chen (2023)
Distress prediction: Intuitively, one would assume that an increase in the cost of debt will have a negative impact on a stock’s riskiness. However, an ML model reveals that this effect is not linear and homogenous; increasing cost of debt is especially detrimental for heavily indebted companies with shorter distance-to-default. Overall, modelling such non-linear patterns together with interaction effects can benefit the prediction of stock price crash risk.
Figure 3: Non-linearity and interaction effects, detected by ML models, for stock distress prediction
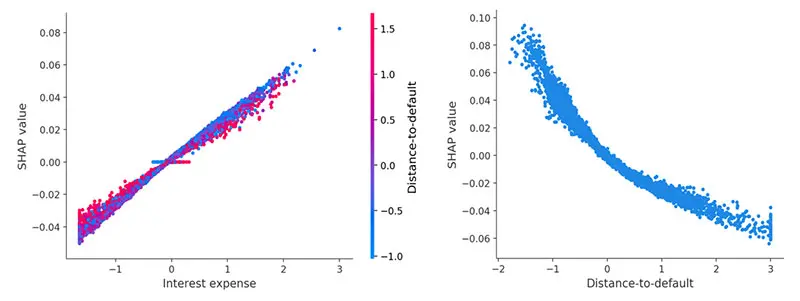
Source: ‘Forecasting stock crash risk with machine learning’, Swinkels and Hoogteijling (2022)
Volatility surfaces: Image recognition is an everyday ML use case, wherein a machine is tasked to identify the main objects in an image. Such use case can readily be translated into a finance application, in which the volatility surface can be seen as an image, with pixel colors as the implied volatility of the options at different moneyness levels and time-to-maturity and convolutional layers to predict stock returns with the data embedded in this image. In this setup, the machine learns the relationship between the option-implied information and the future returns of the corresponding security.
Figure 4: Volatility surface of NVIDIA on 9 October 2023
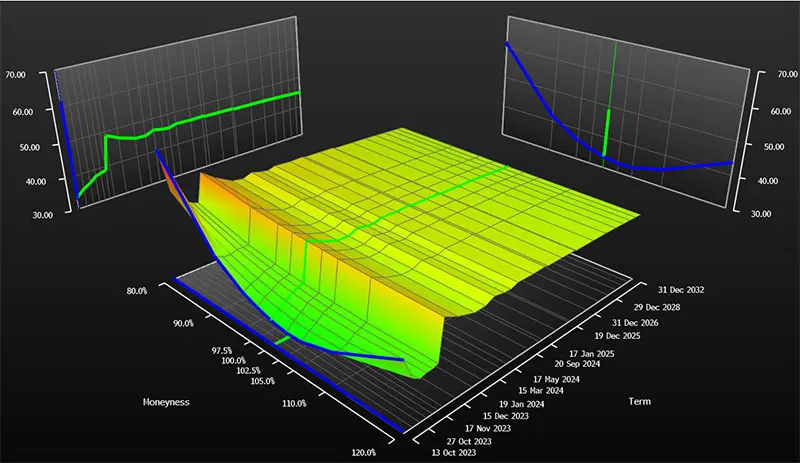
Source: Bloomberg. The Convolutional Neural Networks allow for the extraction of valuable insights from the options volatility surface, enabling predictions on the risk and return associated with stocks.
Get the latest insights
Subscribe to our newsletter for investment updates and expert analysis.
Company clustering and theme identification: Where the above examples are of supervised learning, ML techniques are also well established for unsupervised learning, such as company clustering, where the aim is to form clusters of similar companies based on a large number of characteristics. These clusters can then be used to identify emerging market trends and themes, or for peer group comparison.
Figure 5: Clusters of companies based on company descriptions
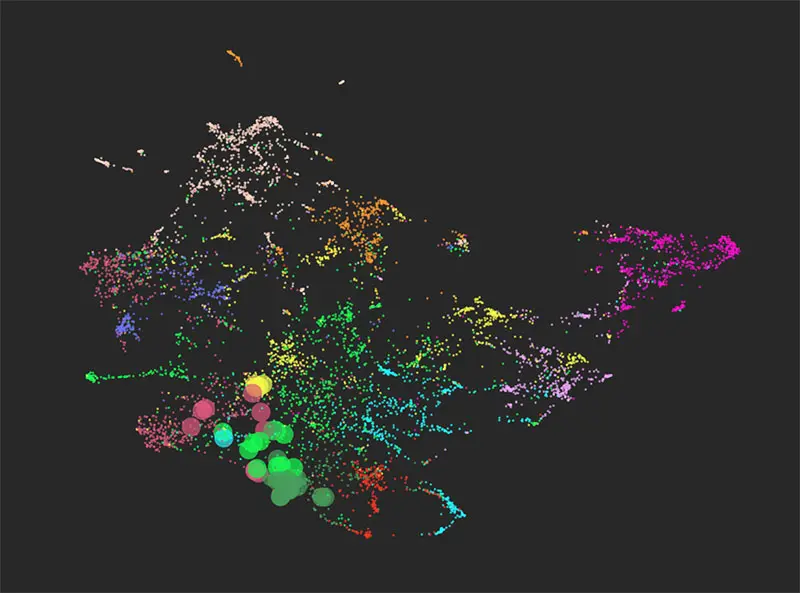
Source: Robeco. In this visualization, every small dot on the graph represents a company, distinguished by different colors based on the GICS (Global Industry Classification Standard) sector they belong to. The dots that are close together indicate groups of companies with similar business descriptions or lines. The larger circles on the graph indicate the top 50 companies that are closely related to the field of “Electric Vehicles”. This graph reveals that the companies involved with “Electric Vehicles” are spread across different GICS sectors including Industrials (light green), Utilities (dark green), Consumer Discretionary (yellow), Information Technology (purple), and Materials (light blue).
Trading: Once a suitable stock has been identified, it becomes crucial to employ the most effective trading strategy. The availability of more independent observations at intraday frequencies allows machines to be furnished with a larger volume of data. This in turn enhances their ability to identify robust patterns in the data, rendering trading as one of the most promising domains for the application of AI in finance. In this way ML can also be extended to other significant use cases, such as broker selection.
The virtue of NLP
Along with the development of ML models, NLP models have made great strides in performing textual analysis and can be applied to sentiment analysis as well as help advance sustainability investing.
Sentiment analysis: The abundance of financial textual data makes it a unique hunting ground for testing NLP models. For instance, sentiment analysis helps to gauge the tone of management speech or company news.
Sustainable investing: Company ESG scores have often been primarily based on self-declared surveys filled by company management. Today, company news (media perception), earnings calls, financial disclosures (management perception), or employee reviews (employees’ perception) can be rich data sources for company profiling, allowing companies to be scored on ESG aspects based on how the media perceives them in each dimension.
The rise of generative AI
The most prominent recent innovations are generative AI models, particularly GPT models in NLP. These are trained on vast text datasets and can interact with the user, although they can make mistakes and still require interactive supervision by a human. In this way humans function as domain experts and validate rather than create the content from scratch.
With the release of generative models tuned for programming, such as Github CoPilot, the machine can act as a sparring partner in coding functions or when translating them from one programming language into another one. GPT models can power chatbots to manage initial customer inquiries, provide automated responses, and even offer basic troubleshooting guidance. They can also function as a helpful research assistant in various ways, generating ideas, conducting basic literature reviews, and summarizing academic papers. They can compare arguments between two papers and answer basic questions, like identifying peer companies of NVIDIA or determining which sectors are impacted by energy price inflation.
Implications and conclusions
The introduction of AI in finance is a significant advancement that can revolutionize various office tasks. While concerns about personnel redundancy are certainly valid, history has shown that humans can adapt and find new avenues for growth and development alongside automation.
Specifically for finance, AI can automate many of the repetitive and labor-intensive processes currently carried out by human practitioners. More intellectually involved aspects may also be speeded up or completely automated by AI, such as finding unique and causal relationships within massive amounts of data or deciding in which asset class to invest, depending on macroeconomic data and market sentiments.
While AI will allow the industry to further evolve, fundamental investing will experience a particularly large disruption – as AI tools become more user friendly, they will allow fundamental investors to acquire the capabilities previously only available to quantitative investors. However, skilled quant investors will have the tools and know-how to customize general AI programs.
For all of today’s practitioners, it will be key to work with AI, whether learning basic skills or building completely custom AIs from the ground up.