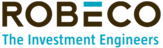
Disclaimer
1. General
Please read this information carefully.
This website is prepared and issued by Robeco Hong Kong Limited ("Robeco"), which is a corporation licensed by the Securities and Futures Commission in Hong Kong to engage in Type 1 (dealing in securities); Type 2 (dealing in futures contracts); Type 4 (advising in securities) and Type 9 (asset management) regulated activities. The Company does not hold client assets and is subject to the licensing condition that it shall seek the SFC’s prior approval before extending services at retail level. This website has not been reviewed by the Securities and Futures Commission or any regulatory authority in Hong Kong.
2. Important risk disclosures
Important risk disclosures Robeco Capital Growth Funds (“the Funds”) are distinguished by their respective specific investment policies or any other specific features. Please read carefully for the risks of the Funds:
Some Funds are subject to investment, market, equities, liquidity, counterparty, securities lending and foreign currency risk and risk associated with investments in small and/or mid-capped companies.
Some Funds are subject to the risks of investing in emerging markets which include political, economic, legal, regulatory, market, settlement, execution, counterparty and currency risks.
Some Funds may invest in China A shares directly through the Qualified Foreign Institutional Investor (“QFII”) scheme and / or RMB Qualified Foreign Institutional Investor (“RQFII”) scheme and / or Stock Connect programmes which may entail additional clearing and settlement, regulatory, operational, counterparty and liquidity risk.
For distributing share classes, some Funds may pay out dividend distributions out of capital. Where distributions are paid out of capital, this amounts to a return or withdrawal of part of your original investment or capital gains attributable to that and may result in an immediate decrease in the net asset value of shares.
Some Funds’ investments maybe concentrated in one region / one country / one sector / around one theme and therefore the value of the Fund may be more volatile and may be subject to concentration risk.
The risk exists that the quantitative techniques used by some Funds may not work and the Funds’ value may be adversely affected.
In addition to investment, market, liquidity, counterparty, securities lending, (reverse) repurchase agreements and foreign currency risk, some Funds are subject to risk associated with fixed income investments like credit risk, interest rate risk, convertible bonds risk, ABS risk and the risk of investments in non-investment grade or unrated securities and the risk of investments made in non-investment grade sovereign securities.
Some Funds can use derivatives extensively. Robeco Global Consumer Trends Equities can use derivatives for hedging and efficient portfolio management. Derivatives exposure may involve higher counterparty, liquidity and valuation risks. In adverse situations, the Funds may suffer significant losses (even a total loss of the Funds’ assets) from its derivative usage.
Robeco European High Yield Bonds is subject to Eurozone risk.
Investors may suffer substantial losses of their investments in the Funds. Investor should not invest in the Funds solely based on the information provided in this document and should read the offering documents (including potential risks involved) for details.
3. Local legal and sales restrictions
The Website is to be accessed by “professional investors” only (as defined in the Securities and Futures Ordinance (Cap.571) and/or the Securities and Futures (Professional Investors) Rules (Cap.571D) under the laws of Hong Kong). The Website is not directed at any person in any jurisdiction where (by reason of that person’s nationality, residence or otherwise) the publication or availability of the Website is prohibited. Persons in respect of whom such prohibitions apply or persons other than those specified above must not access this Website. Persons accessing the Website need to be aware that they are responsible themselves for the compliance with all local rules and regulations. By accessing this Website and any of its pages, you acknowledge your agreement with understanding of the following terms of use and legal information. If you do not agree to the terms and conditions below, do not access this Website or any pages thereof.
The information contained in the Website is being provided for information purposes.
Neither information nor any opinion expressed on the Website constitutes a solicitation, an offer or a recommendation to buy, sell or dispose of any investment, to engage in any other transaction or to provide any investment advice or service. The information contained in the Website does not constitute investment advice or a recommendation and was prepared without regard to the specific objectives, financial situation or needs of any particular person who may receive it. An investment in a Robeco product should only be made after reading the related legal documents such as management regulations, prospectuses, most recent annual and semi-annual reports, which can be all be obtained free of charge at www.robeco.com/hk/en and at the Robeco Hong Kong office.
4. Use of the Website
The information is based on certain assumptions, information and conditions applicable at a certain time and may be subject to change at any time without notice. Robeco aims to provide accurate, complete and up-to-date information, obtained from sources of information believed to be reliable. Persons accessing the Website are responsible for their choice and use of the information.
5. Investment performance
No assurance can be given that the investment objective of any investment products will be achieved. No representation or promise as to the performance of any investment products or the return on an investment is made. The value of your investments may fluctuate. The value of the assets of Robeco investment products may also fluctuate as a result of the investment policy and/or the developments on the financial markets. Results obtained in the past are no guarantee for the future. Past performance, projection, or forecast included in this Website should not be taken as an indication or guarantee of future performance, and no representation or warranty, express or implied, is made regarding future performance. Fund performance figures are based on the month-end trading prices and are calculated on a total return basis with dividends reinvested. Return figures versus the benchmark show the investment management result before management and/or performance fees; the fund returns are with dividends reinvested and based on net asset values with prices and exchange rates of the valuation moment of the benchmark.
Investments involve risks. Past performance is not a guide to future performance. Potential investors should read the terms and conditions contained in the relevant offering documents and in particular the investment policies and the risk factors before any investment decision is made. Investors should ensure they fully understand the risks associated with the fund and should also consider their own investment objective and risk tolerance level. Investors are reminded that the value and income (if any) from shares of the fund may be volatile and could change substantially within a short period of time, and investors may not get back the amount they have invested in the fund. If in doubt, please seek independent financial and professional advice.
6. Third party websites
This website includes material from third parties or links to websites maintained by third parties some of which is supplied by companies that are not affiliated to Robeco. Following links to any other off-site pages or websites of third parties shall be at the own risk of the person following such link. Robeco has not reviewed any of the websites linked to or referred to by the Website and does not endorse or accept any responsibility for their content nor the products, services or other items offered through them. Robeco shall have no liability for any losses or damages arising from the use of or reliance on the information contained on websites of third parties, including, without limitation, any loss of profit or any other direct or indirect damage. Third party off-site pages or websites are provided for informational purposes only.
7. Limitation of liability
Robeco as well as (possible) other suppliers of information to the Website accept no responsibility for the contents of the Website or the information or recommendations contained herein, which moreover may be changed without notice.
Robeco assumes no responsibility for ensuring, and makes no warranty, that the functioning of the Website will be uninterrupted or error-free. Robeco assumes no responsibility for the consequences of e-mail messages regarding a Robeco (transaction) service, which either cannot be received or sent, are damaged, received or sent incorrectly, or not received or sent on time.
Neither will Robeco be liable for any loss or damage that may result from access to and use of the Website.
8. Intellectual property
All copyrights, patents, intellectual and other property, and licenses regarding the information on the Website are held and obtained by Robeco. These rights will not be passed to persons accessing this information.
9. Privacy
Robeco guarantees that the data of persons accessing the Website will be treated confidentially in accordance with prevailing data protection regulations. Such data will not be made available to third parties without the approval of the persons accessing the Website, unless Robeco is legally obliged to do so. Please find more details in our Privacy and Cookie Policy.
10. Applicable law
The Website shall be governed by and construed in accordance with the laws of Hong Kong. All disputes arising out of or in connection with the Website shall be submitted to the exclusive jurisdiction of the courts of Hong Kong.
Please click the “I agree” button if you have read and understood this page and agree to the Disclaimers above and the collection and use of your personal data by Robeco, for the purposes for which such data is collected and used as set out in the Privacy and Cookie Policy, including for the purpose of direct marketing of Robeco products or services. Otherwise, please click “I Disagree” to leave the website.
Our insights
Understanding where markets are going, and the impact that has, is the hardest thing for every investment professional to do. It takes robust research and insight to develop the best understanding, and that’s why we put a lot of effort into it. Because only by sharing knowledge can we all prosper.
0 articles
Focus themes
Content type
Region
Strategies