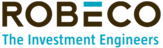
Zugangsbeschränkung / Haftungsausschluss
Die auf diesen Seiten enthaltenen Informationen dienen ausschliesslich Marketingzwecken.
Der Zugang zu den Fonds ist beschränkt auf (i) qualifizierte Anleger gemäss Art. 10 Abs. 3 ff. des Bundesgesetz über kollektive Kapitalanlagen („KAG“), (ii) professionelle Kunden gemäss Art. 4 Abs. 3 und 4 des Bundesgesetz über die Finanzdienstleistungen („FIDLEG“) und (iii) professionelle Kunden gemäss Anhang II der Richtlinie über Märkte für Finanzinstrumente (2014/65/EU; „MiFID II“) mit Sitz in der Europäischen Union oder im Europäischen Wirtschaftsraum mit einer entsprechenden Lizenz zur Erbringung von Vertriebs- / Angebotshandlungen im Zusammenhang mit Finanzinstrumenten oder für (iii) solche, die hiermit aus eigener Initiative entsprechende Informationen zu spezifischen Finanzinstrumenten erfragen und als professionelle Kunden qualifizieren.
Die Fonds haben ihren Sitz in Luxemburg oder den Niederlanden. Die ACOLIN Fund Services AG, Postanschrift: Leutschenbachstrasse 50, CH-8050 Zürich, agiert als Schweizer Vertreter der Fonds. UBS Switzerland AG, Bahnhofstrasse 45, 8001 Zürich, Postanschrift: Europastrasse 2, P.O. Box, CH-8152 Opfikon, fungiert als Schweizer Zahlstelle.
Der Prospekt, die Key Investor Information Documents (KIIDs), die Satzung, die Jahres- und Halbjahresberichte der Fonds sind auf einfache Anfrage hin und kostenlos beim Schweizer Vertreter ACOLIN Fund Services AG erhältlich. Die Prospekte sind auch über die Website www.robeco.com/ch verfügbar.
Einige Fonds, über die Informationen auf dieser Website angezeigt werden, fallen möglicherweise nicht in den Geltungsbereich des KAG und müssen daher nicht über eine entsprechende Genehmigung durch die Eidgenössische Finanzmarktaufsicht FINMA verfügen.
Einige Fonds sind in Ihrem Wohnsitz- / Sitzstaat möglicherweise nicht verfügbar. Bitte überprüfen Sie den Registrierungsstatus in Ihrem jeweiligen Wohnsitz- / Sitzstaat. Um die in Ihrem Land registrierten Produkte anzuzeigen, gehen Sie bitte zur jeweiligen Länderauswahl, die auf dieser Website zu finden ist, und wählen Sie Ihr Wohnsitz- / Sitzstaat aus.
Weder Informationen noch Meinungen auf dieser Website stellen eine Aufforderung, ein Angebot oder eine Empfehlung zum Kauf, Verkauf oder einer anderweitigen Verfügung eines Finanzinstrumentes dar. Die Informationen auf dieser Webseite stellen keine Anlageberatung oder anderweitige Dienstleistung der Robeco Schweiz AG dar. Eine Investition in ein Produkt von Robeco Schweiz AG sollte erst erfolgen, nachdem die entsprechenden rechtlichen Dokumente wie Prospekt, Jahres- und Halbjahresberichte konsultiert wurden.
Durch Klicken auf "Ich stimme zu" bestätigen Sie, dass Sie resp. die von Ihnen vertretene juristische Person in eine der oben genannten Kategorien von Adressaten fallen und dass Sie die Nutzungsbedingungen für diese Website gelesen, verstanden und akzeptiert haben.
Unsere Einblicke
Die Richtung, in die sich die Märkte entwickeln, und die damit verbundenen Auswirkungen zu verstehen, ist die anspruchsvollste Aufgabe jedes Anlageexperten. Um ein bestmögliches Verständnis zu entwickeln, sind leistungsfähiges Research und gründliche Einblicke erforderlich. Deshalb investieren wir sehr viel Zeit darin. Denn nur durch das Teilen von Wissen können wir alle profitieren.
1 Artikel
Fokus-Themen
Inhaltstyp
Region
Strategien